Background & Objectives
Despite rapid advances in Human-computer Interaction (HCI) and relentless endeavors to improve user experience with computer systems, the need for agents to recognize and adapt to the affective state of users has been widely acknowledged. The personality–affect relationship has been actively studied ever since a correlation between the two was proposed in Eysenck’s personality model, which states that Extraversion is accompanied by low cortical arousal and that Neurotics are more sensitive to external stimulation.
Many affective studies have attempted to validate Eysenck’s model, but few have investigated affective correlates of traits other than Extraversion and Neuroticism. Additionally, social psychology studies have examined personality mainly via non-verbal social behavioral cues, but few works have modelled personality traits based on emotional behavior.
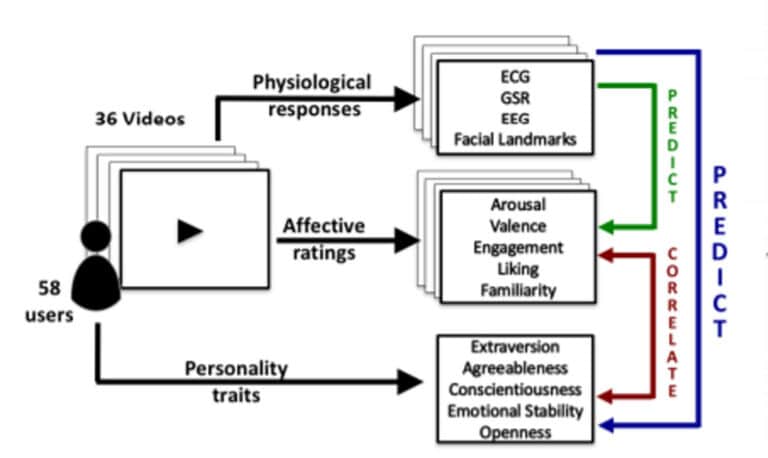
ASCERTAIN Database
This work builds on the ASCERTAIN database, which is a multimodal database for implicit personality and affect recognition. ASCERTAIN contains personality scores and emotional self-ratings of 58 users in addition to their affective physiological responses. More specifically, ASCERTAIN is used to model users’ emotional states and big five personality traits via heart rate, GSR and EEG.
The researchers for this study used this database to understand the relation between emotional attributes and personality traits and characterize both via users’ physiological responses captured through Shimmer’s wearable sensors.
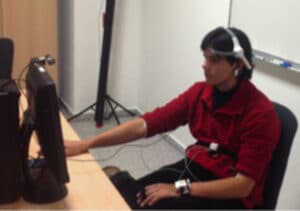
Integrating Shimmer
Integrating the Shimmer3 GSR+ and ECG sensors for this study were very simple. The Shimmer3 GSR+ sensor was placed on the wrist with two electrodes attached to the index and middle fingers on the non-dominant hand of the participants. The Shimmer3 ECG was then mounted on the chest with 5 electrodes attached in the torso region of the body. This setup process takes approximately 5-10 minutes, and once complete, the participant can sit passively and engage with the content in front of them.
For this study, participants engaged with movie scenes as they effectively evoke emotions; the movie genres used were thriller, comedy and horror. Each participant performed the experiment in a session lasting about 90 minutes. The viewing of each movie clip is denoted as a trial; after two practice trials involving clips that were not part of the actual study, users watched movie clips randomly shown in two blocks of 18 trials, with a short break in-between to avoid fatigue. In each trial, a fixation cross was displayed for four seconds followed by clip presentation. After viewing each clip, users self-reported their emotional state in the form of affective ratings within a time limit of 30 seconds. They also completed a personality questionnaire after the experiment.
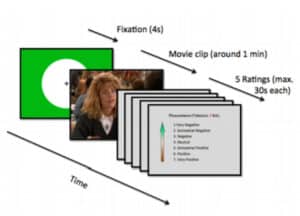
Affective Ratings and Analysis
For each movie clip, the researchers compiled valence (V) and arousal (A) ratings reflecting the user’s affective impression. A 7-point scale was used with a -3 (very negative) to 3 (very positive) scale for V, and a 0 (very boring) to 6 (very exciting) scale for A. Likewise, ratings concerning engagement (Did not pay attention – Totally attentive), liking (I hated it – I loved it) and familiarity (Never seen it before – Remember it very well) were also acquired. For personality scores, participants completed the big-five marker scale (BFMS) questionnaire, which has been used widely for personality recognition.
To examine the relationship between different user ratings, the researchers computed Pearson correlations among self-reported attributes. Focusing on significant correlations, arousal is moderately correlated with engagement, while valence is found to correlate strongly with liking. Additionally, a moderate and significant correlation is noted between engagement and linking, implying that engaging videos are likely to appeal to viewers’ senses.
Considering all movie clips, a significant and moderately negative correlation is noted between extraversion and engagement, implying that introverts were more immersed with emotional clips during the movie-watching task. More information on the analysis can be provided upon request.
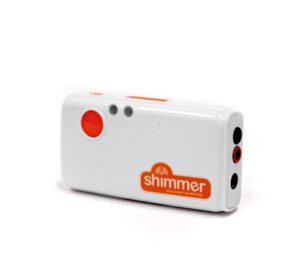
Conclusion and Future Research
This study presents ASCERTAIN, a new multimodal affective database comprising implicit physiological responses of 58 users collected via commercial and wearable GSR, ECG, EEG sensors, and a webcam while viewing emotional movie clips. ASCERTAIN is the first to facilitate study of the relationships among physiological, emotional and personality attributes.
The personality–affect relationship is found to be better characterized via non-linear statistics. Consistent results are obtained when physiological features are employed for analyses in lieu of affective ratings. Additionally, Personality differences are better characterized by analyzing responses to emotionally similar clips, as noted from both correlation and recognition experiments. Finally, RBF SVM achieves the best personality trait recognition, further corroborating a nonlinear emotion–personality relationship.
This study shows ASCERTAIN can facilitate e future AR studies, and spur further examination of the personality–affect relationship. The fact that personality differences are observable from user responses to emotion-wise similar stimuli paves the way for simultaneous emotion and personality profiling.